It’s a simple fact that bears repeating: educators cannot be everywhere at once. However, with the increasing prevalence of AI education technologies (EdTech), it may be possible for educators to extend the scope of their impact both inside and outside the classroom.
If one were to take a step back and observe the overall structure of a modern educational system, they would likely see that teaching strategies and curricula are often based on the concept of scaffolding (1). Broken down into its core components, scaffolding - sometimes described as “I do, we do, you do” teaching - is the practice of gradually relinquishing control of a lesson from an educator to a student; in general, the process begins with formal instruction from a teacher, before moving on to guided practice, and eventually independent learning (2). AI’s place in the education system can perhaps be thought of as somewhere between guided practice and complete student autonomy. In this way, the teacher remains the primary source of information, support, and motivation for the student, while AI acts as a supplementary resource that students can access when needed.
Integrating AI has the potential to create a profound ripple effect throughout the education system. Not only can a greater number of students get access to the educational materials and assistance that they need, but also, by automating less demanding tasks, educators are afforded more time to focus on complex responsibilities and direct student interaction.
AI Chatbots and Tutoring Systems
While teaching is an educator’s principal responsibility, they typically spend many additional hours outside of the classroom answering questions, or addressing academic concerns in order to ensure student comprehension. Specifically in higher education, when courses may enroll hundreds of students per term, the quantity of questions asked can number in the thousands and quickly become difficult to manage (6). Additionally, due to the scale of higher education enrollment, educators are bound to receive many similar if not outright repetitious queries. Although not widely implemented, AI chatbots can prove to be an innovative solution to these challenges, as they can be trained to automatically answer questions regarding coursework, advising, or any other concern students may have.
By employing AI chatbots to answer frequently asked questions, teachers can save countless hours each term, and given the constant availability of AI chatbots, students can receive around the clock assistance in order to become better informed and more readily prepared to meet academic demands.
Due to the relative ubiquity of chatbots today, there is a high possibility that one may already be familiar with their services - most likely in a customer support capacity. However, not all chatbots offer the same level of sophistication, and there is a clear distinction to be drawn between those that use artificial intelligence and those that do not (3). Older chatbots tend to be rule-based, and require exact phrasing from the user in order to match their query with a prewritten answer, whereas AI chatbots (sometimes synonymously referred to as virtual assistants) are capable of making generalizations based on user input and providing answers that are discerned from a given context rather than prewritten (3). AI chatbots generally rely on a combination of natural language processing (NLP) and natural language understanding (NLU) to make sense of the diverse array of questions and commands that may be entered by a user (3).
NLP and NLU are two sides of the same coin, as they both handle the interpretation of human language albeit from different perspectives (4). NLP converts the diverse and irregular nature of human language into a standardized structure, so that an algorithm can better make sense of the individual components of a sentence, and the grammatical structure therein (4). NLU derives user intentions and the meaning of language from context, by logging the conversation and referring new statements back to the origin; this is especially important since the meaning of words and phrases are apt to shift depending on the context in which they are used (4). In short, NLP handles what is literally being said, while NLU handles what is meant (4).
How effectively an AI chatbot performs is largely dependent on the quality and quantity of data it is trained with. More sophisticated AI chatbots, like OpenAI’s GPT-3 system, use deep learning and monumental amounts of data - in the case of GPT-3, hundreds of billions of words largely amassed from CommonCrawl and other internet archives - in order to generate informed, human-like responses (5). The quality of output generated by the likes of GPT-3 can be truly impressive; however, for the purposes of aiding in academic advising or coursework, the training data need not be so comprehensive, since the chatbot will generally be operating in a much narrower field.
In an experimental study conducted by Ashok Goel, professor of Interactive Computing at Georgia Tech University, an AI chatbot was trained off of a relatively slight dataset consisting of forum posts from previous semesters of Goel’s course, and tasked with answering student questions regarding the course syllabus and assignments (6). The quality of text responses generated by the AI were sufficiently human-like so as to be almost unanimously mistaken for a human teaching assistant (6). While the initial study was carried out with the purpose of testing the interactive quality of an AI chatbot, the results and subsequent implementation of Goel’s chatbot showed how efficiently
AI chatbots can automate administrative tasks, free up time for teachers and teaching assistants, and provide rapid responses to students (6).
In order to guarantee accurate responses, Goel’s chatbot was programmed to include an accuracy threshold; simply put, if the chatbot is not 97 percent confident in its answer, it is not allowed to answer (6). The application of an accuracy threshold ensures that the vast majority of common or simplistic questions are answered automatically, while the most complex or demanding questions are diverted to teachers and teaching assistants, in effect significantly narrowing educators’ workloads.
When combined with knowledge of a specific domain and one or more pedagogical models, AI chatbots can become more akin to full-fledged intelligent tutoring systems (ITS) that are capable of not only answering questions, but also providing supplementary resources, and tailored remediation strategies. In these cases, it might be more accurate to think of them less as AI chatbots, and more as ITS with added chatbot capabilities, as they are less concerned with replicating human interaction, and more concerned with facilitating academic growth.
One of the more interesting examples of a chatbot enabled ITS is Korbit AI, a platform designed to teach college-level data science. Similar to other chatbots, Korbit’s interface takes the form of an online chat log. However, instead of purely text-based interaction, Korbit intersperses short lecture videos that are succeeded by automatically generated questions. Once presented with a question, the student may choose to skip it, request assistance, or attempt to answer it. If the solution is classified as incorrect, or the student asks for help, Korbit will implement one of several intervention strategies that may include mathematical hints, written clarification, or multiple choice options (7).
What intervention strategy Korbit deems best is not random; in fact, it has a firm basis in a well established pedagogical theory known as the Zone of Proximal Development (7). The Zone of Proximal Development is defined as “the difference between what a learner can do without help and what [they] can achieve with guidance and encouragement from a skilled partner” (8). In line with this theory, it is imperative that Korbit is made aware of any prior instances where the student required help to arrive at the correct answer, and it achieves this by referring back to the student’s performance on previous questions (7). If, for example, the student had repeatedly answered questions incorrectly, Korbit might offer a more intensive intervention strategy (7). Korbit’s basis in AI chatbot technology becomes apparent during this process, as it refers back to previous interactions with the student, similarly to how an AI chatbot uses previous messages to provide context for current conversations.
AI Language Assessments
It is well documented that in recent years, particularly due to the pandemic, reading proficiency has dramatically decreased amongst elementary school students (9). The exact reasoning is difficult to pin down as every child learns at a different pace and in a different way, but regardless, the resumption of in-person instruction has revealed stagnated or otherwise diminished reading comprehension and oral reading fluency skills (9). As a result, many educators - especially those at the kindergarten, first, and second grade level - have found themselves allocating a significant portion of their school days to teaching low-level phonics, instead of advancing to more demanding or age-appropriate learning material (9). Reading fluency is the bedrock upon which every other aspect of academic progress is built; as such, it would be ill advised to skip or rush through reading fluency lessons. However, it is possible that some of the strain on teachers can be relieved, and the process made more efficient by automating reading fluency assessments with the aid of AI reading tutors (10).
Amira Learning, an EdTech company conceived at Carnegie Mellon University, has proposed that their flagship program, Amira and the Storycraft, can save teachers up to ninety hours a year by automating reading assessments and other associated administrative tasks (10). Traditionally, the most popular means of testing reading proficiency in the classroom is through guided reading exercises, in which an instructor listens to students read book passages aloud and offers assistance as needed. Due to the differentiated nature of this assessment, it can be an extensive process. Amira replicates guided reading exercises by using AI to recommend short stories for students to read aloud, and assesses their proficiency by listening to their pronunciation, and keeping ongoing records of their progress (11).
Amira makes use of automated speech recognition (ASR), a subfield of machine learning that gives users the ability to communicate with technology using their voices (11). By breaking down spoken dialogue into distinct units of sound called phonemes and analyzing their sequence, ASR algorithms are able to make sense of entire words, or in the case of more advanced continuous speech recognition algorithms, entire sentences (12). Amira is an example of the latter, as it is able to listen to students read entire story passages uninterrupted, only breaking the flow to provide feedback. Fundamentally, Amira is using a comparison model that contrasts the reader’s proficiency with that of fluent speakers, emphasizing “specific syntactic and lexical features of text that can be used to predict fluency and comprehension” (11). The dataset used for training was gathered over an extensive period and consists of many audio files of language spoken by both children and adults, with differing levels of reading proficiency so as to account for variation in input (11) (13).
While the technologies used in AI tutoring services tend to be fairly novel, the content - i.e. coursework, teaching strategies, etc. - is often firmly rooted in time-tested pedagogical models.
In Amira’s case, the lessons are all designed to reflect the 5 Pillars of Literacy: phonemic awareness, phonics, fluency, vocabulary, and comprehension (11). If a student struggles at any point during their reading assessment, Amira is designed to provide one of several “micro-interventions” (14). For example, if a student struggled to pronounce “spread”, Amira might suggest “red” as a rhyming word, thereby implementing a strategy often practiced when teaching phonemic awareness (14).
The data collected from student reading assessments serves dual purposes; in addition to providing a basis from which to compare student reading proficiency, it also informs Amira’s recommendation algorithm. Once a student completes a reading assessment, Amira provides them with a selection of five stories to read, each of which is specially selected based on the level of proficiency exhibited. More specifically, the recommendations are aimed at facilitating a 90% comprehension and a 10% error rate, so as to continuously expose readers to unfamiliar words or ideas. Additionally, Amira takes into account a reader’s schema - that is, their background knowledge - as students are likely to perform better when reading stories that incorporate recognizable concepts, nouns, and word associations. If, for instance, a reader performed consistently well when reading stories about dinosaurs, Amira might challenge a reader by recommending stories outside of their comfort zone (15).
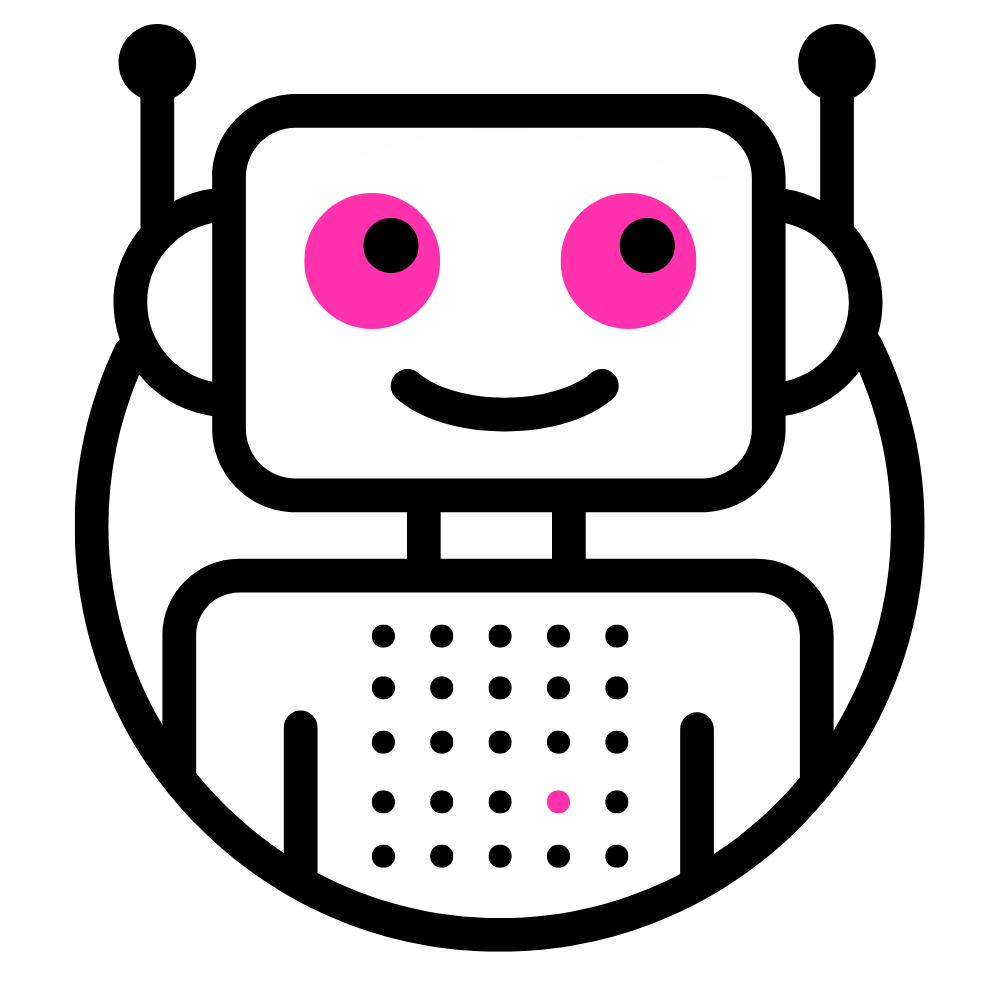
Need help answering the following questions?
Talk it over with our AI bot Xybo.
AI Grammar Assistance and Feedback
Numerous surveys and teacher testimonials suggest that educators spend, on average, between five and ten hours per week grading student work and providing feedback (16). However, not all feedback is likely to result in the same level of academic improvement. In a meta-analysis of the efficacy of various forms of teacher-student feedback, it was revealed that three of the most important criteria when providing meaningful feedback to students are depth, specificity, and immediacy (17). Unfortunately, short of full scale differentiated instruction, it can be difficult to provide adequate feedback to every student, especially when one considers the diversity of individual needs present in each classroom. AI can provide a solution; by automating low-level feedback and error correction, educators can devote more time to providing structural, content-specific feedback to students. As an example of a fully-realized automated feedback mechanism, consider the breadth of feedback delivered by modern AI grammar assistants.
There are a number of grammatical assistants currently leveraging AI such as Trinka, and Linguix, that specialize in technical and professional writing respectively, but undoubtedly the most widely used by students and educators is Grammarly. Although Grammarly has long surpassed its own modest origins as a means of checking spelling and punctuation, it might not be incorrect to classify it as a logical evolution of the spell checker. Whereas a spell checker is limited to identifying spelling mistakes in typed work, Grammarly goes many steps further by implementing AI to highlight the misuse of punctuation and grammar, as well as the use of other more abstract compositional concepts like wordiness, hedging, vagueness, formality, and specific tone indicators (18).
While it may seem free form and highly irregular on the surface, written language actually follows fairly recognizable patterns - i.e. grammatical rules (19). When fed enough data
Machine learning algorithms perform exceedingly well at identifying patterns and highlighting the underlying grammatical structure of written language.
Once again, the quality of data used in the training process is paramount. Grammarly was trained on an extensive corpus of texts that exhibit both grammatical perfection and naturally occurring grammatical errors, in order to learn how to differentiate between the two. Once able to fully grasp a grammatical concept, and the multitudinous variations possible, Grammarly can be applied to pinpoint errors or make suggestions to students’ written work. Additionally, Grammarly is constantly being revised via direct feedback from the user. If a suggestion is deemed unfavorable, the user is able to disregard it, which in turn informs the algorithm which situations its suggestions are optimally applied in, and what other suggestions might be more helpful in the future (20).
Although AI grammar assistants are most commonly used to remediate minor mistakes, the most advanced iterations are rapidly approaching a level of nuance that is nearly on par with human educators. Referring back to the aforementioned criteria for meaningful feedback - depth, specificity, and immediacy - Grammarly performs remarkably well in all areas. Grammarly not only provides feedback on a wide range of grammatical parameters, but also goes into fairly thorough detail as to how to remediate specific syntactical errors, even going so far as to automatically rephrase sections of students’ writing (21). However, perhaps most importantly, Grammarly provides feedback instantaneously, thus affording students the time necessary to act on any proposed suggestions.
AI Homework Help
AI education technologies tend to exhibit immense scalability, and can empower a relatively small number of educators to reach a disproportionately large number of students. For evidence of this fact, one might consider the AI integration of Indonesian EdTech startup CoLearn. Indonesia is the fourth most populous country in the world, and in line with this metric, has the fourth largest education system in the world (22). However, it would be erroneous to conflate scale with status, as Indonesia’s education system suffers from pervasive challenges - namely low PISA (Program for International Student Assessment) scores, and low tertiary education attainment rates - that cause it to rank lower than many other Southeast Asian countries in terms of academic achievement (23). The challenges that Indonesia faces are, at least in part, unique to the country, as its combination of a decentralized education system, geographic separation, and regional diversity have made enforcing standards of curricula extremely difficult (24). Perhaps as a result of these difficulties, Indonesia has developed a deeply embedded tutoring culture, and it is not uncommon for students to spend two or three hours attending extracurricular tutoring centers to supplement their classroom education (25). Abhay Saboo, co-founder and CEO of CoLearn, believes that
AI can be used in tandem with existing tutoring infrastructure to provide more streamlined and efficient academic assistance (25).
As Saboo explains, many students who attend in-person tutoring centers in Indonesia - often traveling great distances to reach them - are simply looking for answers to homework questions that their parents or teachers can not answer. “It's time consuming, it's expensive, it's inconvenient…what we saw is that [that] whole 3 or 4 hours can easily be replaced with technology” (25). In an effort to provide students with a more convenient and accessible supplementary education, CoLearn has spent the past three years curating a vast repository of video lessons recorded by hundreds of tutors across Indonesia, covering a diverse array of math, chemistry, and physics topics (26). As it stands, CoLearn currently hosts over 300,000 video lessons that have been screened for accuracy, tone, and style in order to build a high quality, standardized tutoring database (27). Taken alone, the breadth of content is impressive, however, AI integration has made accessing said content exponentially more efficient.
CoLearn’s “Ask” feature makes use of a student’s smartphone camera to scan and upload photos of homework problems before matching them with a relevant video from CoLearn’s repository (26). In this instance, machine learning is primarily used as a means of converting the captured images into a format that is readable by the video selection algorithm. Once a photo of a math or science problem is taken through the CoLearn app, it uses a combination of computer vision and NLP to identify specific written features and comprehend what is being asked (22). For CoLearn’s purposes, the degree of computer vision employed does not need to be as advanced as that which, say, recognizes human faces; instead, CoLearn is primarily reliant on optical character recognition (OCR), the subcategory of computer vision that handles text recognition (28). Although CoLearn is currently limited to scanning typed text, similar applications have found success in the application of computer vision for analyzing handwritten problems as well (29).
CoLearn’s AI integration is rather innocuous, yet it is consistent with the idea that AI can extend educators’ reach far beyond the walls of the classroom. According to Saboo, prior to the implementation of AI, “[CoLearn was] able to answer maybe a few 100 questions in a day, now [it is] able to answer a few 100,000 questions in a day” (25). In scaling up the operation, it is important to note that the human connection between teacher and student is not lost, as all the actual tutoring is being performed by qualified educators, while AI is used primarily as an intermediary between student and content. Furthermore, the human element is reflected in the delivery of CoLearn’s video lessons; rather than providing students with a brief, direct answer to their questions - something that most calculators can reasonably handle - CoLearn’s video lessons are designed to replicate the classroom experience by succinctly explaining the steps required to arrive at a solution (25).
As technology becomes more advanced, there is a consistent cycle where that which was novel becomes commonplace, often for the simple reason that it facilitates greater efficiency or otherwise eases the burden previously allotted to human workers. In much the same way, AI is becoming an essential asset to the modern education system by automating tasks, extending educators’ reach, and providing actionable feedback to students. For AI to be effective in its role, it does not need to dramatically change the existing landscape or paradigm. The automation of specific exercises or the subtle advancement of existing resources can provide immense benefit to the educational system without sacrificing the human connection that is so imperative for academic growth.
SOURCES:
What is Scaffolding in Education? (2022, February 23). GCU. Retrieved July 8, 2022 from, https://www.gcu.edu/blog/teaching-school-administration/what-scaffolding-education#:~:text=Scaffolding%20refers%20to%20a%20method,how%20to%20solve%20a%20problem
I Do, We Do, You Do. (n.d.). Strategies. Retrieved July 8, 2022 from, https://strategiesforspecialinterventions.weebly.com/i-do-we-do-you-do.html
How do Chatbots Work? A Guide to Chatbot Architecture. (n.d.). Maruti techlabs. Retrieved July 8, 2022 from, https://marutitech.com/chatbots-work-guide-chatbot-architecture/
Kidd, C., Saxena, B. (2021, May 13). NLP vs NLU: What’s The Difference? BMC. https://www.bmc.com/blogs/nlu-vs-nlp-natural-language-understanding-processing/
Constantine, W., Dhillon, D. (2021, June 23). Modern AI Text Generation: An Exploration of GPT-3, Wu Dao 2.0 & Other NLP Advances. Xyonix. https://www.xyonix.com/blog/modern-ai-text-generation-exploration-gpt-3-wu-dao-2-nlp-advances
McFarland, M. (2016, May 11). What happened when a professor built a chatbot to be his teaching assistant. The Washington Post. https://www.washingtonpost.com/news/innovations/wp/2016/05/11/this-professor-stunned-his-students-when-he-revealed-the-secret-identity-of-his-teaching-assistant/
Kochmar, E., Do Vu, D., Belfer, R., Gupta, V., Vlad Serban, I., Pineau, J. (2020, June 30). Automated Personalized Feedback Improves Learning Gains in An Intelligent Tutoring System. Lecture Notes in Computer Science, 12164. https://doi.org/10.1007/978-3-030-52240-7_26
Mcleod, S. A. (2019). The Zone of Proximal Development and Scaffolding. Simply Psychology. https://www.simplypsychology.org/Zone-of-Proximal-Development.html
Barshay, J., Flynn, H., Sheasley, C., Richman, T., Bazzaz, D., Griesbach, R. (2021, November 10). America’s reading problem: Scores were dropping even before the pandemic. The Hechinger Report. https://hechingerreport.org/americas-reading-problem-scores-were-dropping-even-before-the-pandemic/
Wang, J. (2022, February 7). Amira Learning Co-Founder, Mark Angel, on the Future of AI in Education. Owl Ventures. https://owlvc.com/insights-the-future-of-ai-in-education.php
Amira Learning. (2020). Research Foundations: Evidence Base. Houghton Mifflin Harcourt. https://www.readwithamira.com/assets/documents/Amira_Learning_Research_Foundations_Nov2020.pdf
Foster, K. (2021, November 9). What is ASR? A Comprehensive Overview of Automatic Speech Recognition Technology. AssemblyAI. https://www.assemblyai.com/blog/what-is-asr/
Erickson, S. (n.d.). How does Amira handle accents or speech impairments? Amira Learning. https://support.amiralearning.com/en/articles/5460718-how-does-amira-handle-accents-or-speech-impairments
Wutzke, L. (n.d.). How does Amira help students learn how to read? Amira Learning. Retrieved July 8, 2022 from, https://support.amiralearning.com/en/articles/5337390-how-does-amira-help-students-learn-how-to-read
Wutzke, L. (n.d.). How does Amira choose the stories students read? Amira Learning. Retrieved July 8, 2022 from, https://support.amiralearning.com/en/articles/6260453-how-does-amira-choose-the-stories-students-read
Hardison, H. (2022, April 2022). How Teachers Spend Their Time: A Breakdown. EducationWeek. https://www.edweek.org/teaching-learning/how-teachers-spend-their-time-a-breakdown/2022/04
Wisniewski, B., Zierer, K., Hattie, J. (2020, January 22). The Power of Feedback Revisited: A Meta-Analysis of Educational Feedback Research. Frontiers in Psychology. https://doi.org/10.3389/fpsyg.2019.03087
The Grammarly Editor: New Look, More Suggestions, Better Writing. (2019, July 16). Grammarly. Retrieved July 8, 2022 from, https://www.grammarly.com/blog/better-writing-with-grammarly/#:~:text=The%20more%20Grammarly%20knows%20about,for%20that%20type%20of%20reader
Dickson, Ben. (2019, October 17). Grammarly AI: The sweet spot of deep learning and natural language processing. TechTalks. https://bdtechtalks.com/2019/10/17/grammarly-ai-assistant-grammar-checker/
How We Use AI to Enhance Your Writing. (2019, May 17). Grammarly. Retrieved July 8, 2022 from, https://www.grammarly.com/blog/how-grammarly-uses-ai/#:~:text=Grammarly%27s%20AI%20system%20combines%20machine,even%20paragraphs%20or%20full%20texts
Transform Whole Sentences for Clarity with Our New Writing Suggestions. (2022, June 24). Grammarly. Retrieved July 8, 2022 from, https://www.grammarly.com/blog/transform-whole-sentences-clarity-suggestions/
Dietmar, J. (2021, July 19). Three Ways Edtech Platforms Can Use AI To Deliver Effective Learning Experiences. Forbes. https://www.forbes.com/sites/forbestechcouncil/2021/07/19/three-ways-edtech-platforms-can-use-ai-to-deliver-effective-learning-experiences/?sh=53b5f9524f98
OECD. (2021). Indonesia: Overview of the education system (EAG 2021). Education GPS. https://gpseducation.oecd.org/CountryProfile?primaryCountry=IDN&treshold=10&topic=EO
Varagur, K. (2019, December 16). Indonesia Education Lags Behind Region. VOA. https://www.voanews.com/a/east-asia-pacific_indonesia-education-lags-behind-region/6181132.html
Asokan, A. (Host). (2021). CoLearn (02). [Audio Podcast Episode]. In The AI-Native Podcast. blox.ai. https://getblox.ai/podcasts/colearn-edtech-ai-technology/
Shu, C. (2021, April 19). Indonesian edtech CoLearn gets $10M Series A led by Alpha Wave Incubation and GSV Ventures. Techcrunch. https://techcrunch.com/2021/04/19/indonesian-edtech-colearn-gets-10m-series-a-led-by-alpha-wave-incubation-and-gsv-ventures/
CoLearn. (n.d.). How to Ask on CoLearn. CoLearn. Retrieved July 8, 2022 from, https://colearn.id/tanya
Bhagtani, A. (2021, March 2021). Computer Vision: Intelligent Automation that Sees. Automation Anywhere. https://www.automationanywhere.com/company/blog/rpa-thought-leadership/computer-vision-intelligent-automation-that-sees#:~:text=Computer%20vision%20versus%20OCR%20technology&text=OCR%20is%20a%20subset%20of,limited%20foray%20into%20computer%20vision
O’Keefe, S. (2016, September 21). Former Battlefield finalist Photomath app can now solve your handwritten math problems. Techcrunch. https://techcrunch.com/2016/09/21/former-battlefield-finalist-photomaths-app-can-now-solve-your-handwritten-math-problems/