According to a 2021 Mckinsey report, the insurance industry “is on the verge of a seismic, tech-driven shift. [The integration of artificial intelligence] could increase productivity in insurance processes and reduce operational expenses by up to 40% by 2030.” (1) These predictions, coupled with the digitalization of insurance companies and the emergence of AI-driven “insurtech” startups, paint a clear picture of the insurance industry’s future (2). In light of these facts, you may be interested in investing in your own AI capabilities to stay ahead of the curve. However, with the seemingly endless possibilities of AI, it can be difficult to know where to start.
For insurance companies with burgeoning AI capabilities, natural language processing (NLP) solutions are a great place to start. In short, NLP is a category of AI concerned with giving computers the ability to interpret and derive meaning from human language. NLP enables a wide variety of solutions, but is often used to classify text and extracting key insights from text and transcribed audio data.
What makes NLP such a valuable asset to the insurance industry?
For starters, NLP has steadily advanced in recent years, and will continue improving for a long time to come. Additionally, with the advent of transfer learning techniques, NLP solutions are becoming cheaper to develop, and easier to transfer to different aspects of your organization (3). This second point is especially important; since so much of an insurance employee’s time is spent analyzing data and classifying text, investing in a system that can automatically perform these duties in several different contexts can increase your organization’s efficiency across the board. Let’s consider a few specific use cases.
Automating Underwriting and Claims Management
If you were asked to picture an insurance underwriter you might envision someone in formal attire, sitting behind a desk with stacks and stacks of paperwork piled up around them. Although nowadays many of the documents an underwriter requires are digitized, the image of an underwriter bogged down by mounds of unstructured data is not far from reality. In order to evaluate risk and make informed insurance eligibility decisions, underwriters must comb through large amounts of insurance applications, policy documents, and customer communications. With so much data to parse through, it can be difficult for underwriters to maintain a consistently high level of accuracy and efficiency.
While all this unstructured data may be a pain-point for your employees, it’s fertile ground for training and deploying NLP models. When properly trained, NLP algorithms are capable of adding some much needed structure to previously unstructured data sources. Through a process known as text mining, NLP can comb through vast quantities of data and pull out the most relevant information. Similarly, with named entity recognition, NLP algorithms can automatically extract specific items like names, dates, locations, and medical diagnoses (4).
Part of what makes NLP so powerful is that it is not limited to analyzing digital data.
When combined with optical character recognition (OCR), NLP is capable of understanding handwritten text, thus allowing anything from doctors notes to on-site accident reports to be more easily incorporated into an underwriter's calculation of risk (5).
With all this information close at hand, underwriters can much more quickly approve customers and decide the terms of their plans.
The difficulties associated with unstructured data are not unique to underwriting. Walk through any department in your organization and you’ll notice that a vast majority of your employees spend a portion of their day handling unstructured data. In fact, IBM estimates that up to 30% of a knowledge worker’s day is spent searching for the information required to complete their job (6). Investing in a pilot project like automated text classification can have a ripple effect throughout your entire organization, as it could be leveraged across most, if not all, of your departments.
For instance, NLP and text classification can help automate claims management. With NLP, important details such as the type of claim, date of the incident, or the policyholder’s personal information can automatically be extracted from claims and entered into your company’s database. Additionally, the text of claims can be analyzed for key terms that indicate the potential cause of loss. For example, an NLP algorithm could be trained to identify concepts like “car accident” or “flood damage” and classify the claims accordingly.
NLP can also help streamline more drawn-out processes like claims triage. Claims triage is often likened to shooting a moving target, as insurance claims are constantly being updated as new information becomes available. This can pose a problem when attempting to accurately sort claims by urgency, as claims that seem low-cost on cursory review may dramatically increase in cost over time. NLP, working in tandem with predictive analytics, can provide a solution by helping forecast the size of claims. By evaluating incoming data for language characteristic of claims with large costs or uncertain outcomes, the algorithm could inform adjusters of possible changes in claim size in real-time. From there, adjusters could fast-track claims likely to be smaller, or prioritize more urgent, time-sensitive claims (7).
Reducing Churn and Improving Customer Service
A 2020 survey released by Callminer, a global leader in customer analytics, revealed that customer churn in the insurance industry is on the rise. In 2020 alone, customer churn cost insurance companies $2.59 billion, and many additional policyholders reported planning to switch insurance providers in the subsequent 12 months (8). While some amount of customer churn is unavoidable, a significant number of customers reported terminating their plans for reasons that could be largely prevented if given the proper attention.
To prevent customers from churning, you must be able to accurately predict which customers are likely to terminate their plans in the first place. Examples of key concerns include:
Who is likely to churn?
Why are they planning to churn?
What can you do to prevent them from churning?
In theory, customers on the verge of churning exhibit common behaviors, or reveal underlying sentiments, that are consistent with others who have terminated their plans. Some of these behaviors can be found in structured data sources like insurance plans, payment histories, or claims records. However, a significant portion are hidden in more analytically-demanding, and unstructured natural language sources (9). These sources - email correspondence, call transcripts, social media posts, etc. - offer a wealth of information that NLP algorithms can use to identify churn behaviors before it’s too late.
For example, during a call with a representative, a customer might briefly raise concern about the size of their deductible. If this concern is not resolved in the moment, it could get buried and forgotten in the stacks of daily call data. However, an NLP model could immediately mark complaints of this nature as needing remediation, ensuring that the customer gets the help they need.
Although analysis of this sort can be helpful in cases where a customer directly relates their concerns, a lot of feelings may be expressed indirectly, and this data is equally valuable. With the help of sentiment analysis, NLP can uncover more subtle emotional clues in customer interactions. The most common type of sentiment analysis focuses on identifying positive, neutral, or negative sentiment in text; however, more nuanced models capable of recognizing specific moods like happiness, anger, urgency, or even interest level have been developed in recent years (10).
When aiming to identify specific reasons for customer churn, you might consider implementing aspect-based sentiment analysis (ASBA). ASBA mines customer data for correlations between a particular sentiment, and a specific “aspect” of your organization (11). For instance, you might run ASBA on a sample of customer support conversations and discover that customer’s frequently exhibit negative sentiment when discussing the length of hold times. With ASBA, you can more accurately pinpoint areas of customer dissatisfaction, which in turn may reveal reasons customers have for churning.
In many ways, reducing customer churn goes hand in hand with improving customer service. Although perhaps not the leading cause of customer churn, poor customer service is an often-reported reason for customer dissatisfaction in the insurance industry (8). However, poor customer service is not inherent to the industry; it is often a symptom of over-burdened employees. When customer service representatives are swamped with calls and service requests, it’s nearly impossible to give each customer the attention they need.
NLP can improve customer service by providing round-the-clock access to automated self-service channels - namely, chatbots. Chatbots are most effective when trained to answer common queries, such as those related to making a payment, resolving a technical issue, or inquiring about a product or service. Although older generations of chatbots were limited to matching predefined questions with prewritten answers, modern NLP-powered chatbots can provide personalized feedback and expand upon topics in much greater detail. Using chatbots to handle repetitive customer service requests can free up time for your “super agents” - that is, representatives that are driven, empathetic, and exceptionally capable - to tackle more complex problems (8).
Setting up automated self-service channels can also inform customer churn analysis. By opening up additional channels for customer interaction, you are effectively creating new avenues through which to receive customer feedback. This feedback can in turn be mined for valuable sentiment and opinion data, and used to identify your customers’ chief complaints and potential reasons for churning. With this knowledge in hand, you can take a proactive approach and attempt to resolve your customers’ concerns before they decide to terminate their plans. Taking the time to ensure customer satisfaction is almost always going to be beneficial, as it costs much more to acquire new customers than it does to retain existing ones.
Detecting Fraud
A conservative estimate places yearly losses due to insurance fraud at $80 billion per year, while others, like the Coalition Against Insurance Fraud have stated that the true cost is closer to $308.6 billion per year (12). In either case, insurance fraud can be detrimental to your company’s financial stability. The burden of fraud often ends up trickling down to your loyal customers, causing their premiums to increase by an average of $400 to $700 each year, and perhaps even giving them a negative impression of your business (13). Unfortunately, due to the high volume of insurance claims filed each day, it’s likely that many signs of fraudulent activity slip by undetected.
NLP can help in a few ways. As previously mentioned, NLP and text mining can unlock details hidden in unstructured data. Nowhere is this more vital than in insurance claims - where adjuster notes, social media posts, emails and other unstructured sources are relied upon for determining the legitimacy of claims. Traditionally, unstructured sources would require a human touch to make sense of, but as AI powered NLP technologies like ChatGPT have improved, they have become remarkably capable of handling conversational language and ambiguity. The more policyholder data you have access to, the more information you have to mine for suspicious behavior that may be indicative of fraud.
When trained on enough historical instances of fraud, NLP algorithms can efficiently identify discrepancies in insurance claims. For example, a customer might exaggerate the cost of a stolen stereo, and inflate the value by a few hundred dollars despite receipts or credit card statements proving otherwise. Likewise, a customer applying for workers compensation might claim to have an injury that is contrary to that which was included in their official medical reports. With NLP, inconsistencies of this nature can be systematically flagged for review.
NLP can also flag insurance claims that are suspiciously consistent. More specifically,
claims filed by multiple claimants in different locations that contain similar keywords, phrases, or accident descriptions can be flagged for signs of organized fraud (14).
In this context, you might think of the NLP algorithm as a sort of advanced plagiarism checker, consistently comparing incoming claims to previously filed claims. Without an NLP algorithm, this process would be impossible; to even approach the accuracy of an NLP model, an SIU professional would need to know the content of an unrealistically large number of insurance claims.
As a final point, NLP can help identify more localized, customer-specific instances of fraud. By analyzing a policyholder’s claim history, NLP can gain a baseline understanding of their behavior, and draw attention to any suspicious or inconsistent activity. Aside from the obvious benefit of reducing fraudulent claims payouts, being able to quickly and accurately detect fraud can prevent legitimate claims from being delayed.
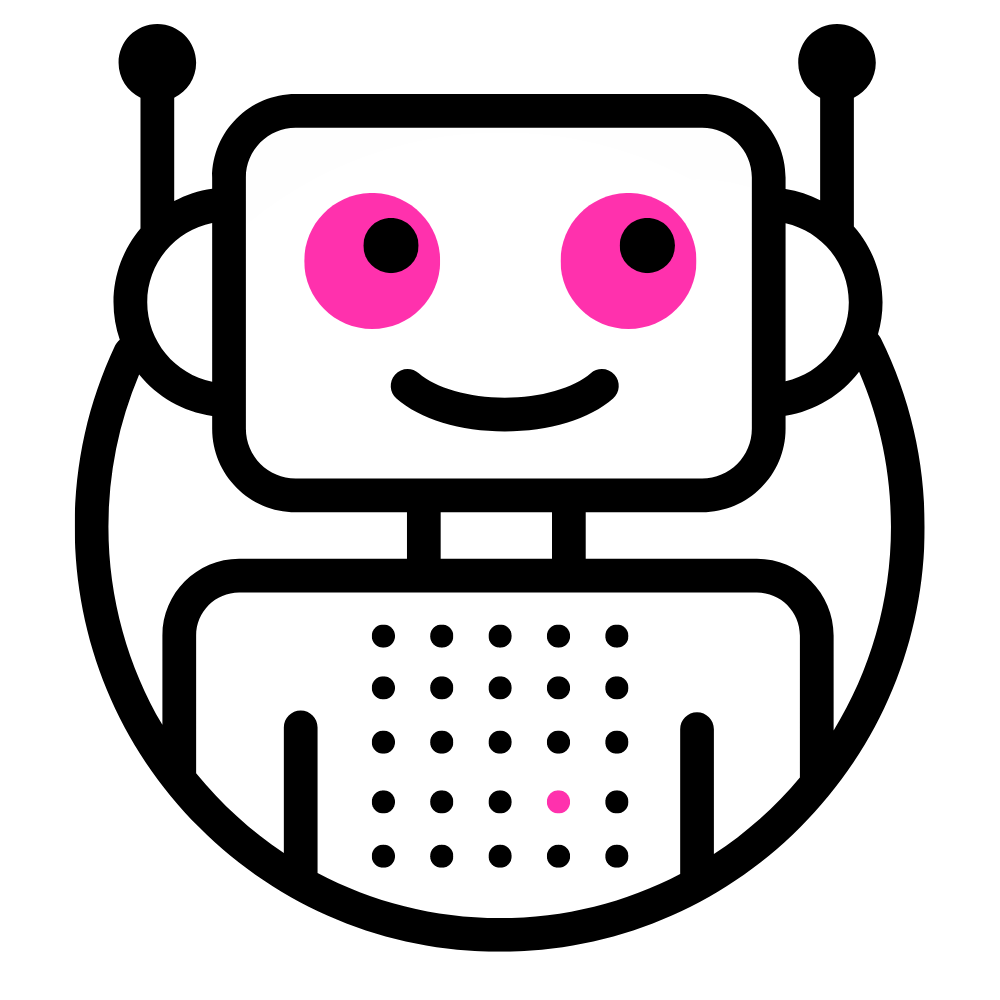
Need help answering the following questions?
Talk it over with our AI bot Xybo.
What Are Some Best Practices For Implementing NLP?
1. Invest in high-quality training data: High-quality NLP models require high-quality training data. Investing in large quantities of diverse, and well-labeled data can dramatically improve the efficacy of your models. Although the insurance industry has ample amounts of data, you’ll likely need to transform it into a machine readable format before you can use it with your models. You might begin by converting digital data like emails and call transcripts before moving on to more demanding data sources like handwritten documents or audio recordings - these will require competency in optical character recognition (OCR) and automated speech recognition (ASR) respectively.
2. Collaborate with domain experts: NLP is ultimately a tool in service of the user. As such, it’s important to collaborate with domain experts such as underwriters, claims adjusters, and fraud analysts, to ensure that your models are designed to meet their specific needs. Although they may not speak in terms of AI, these domain experts can likely use their specialized knowledge to identify domain-specific tasks that would benefit from automation.
3. Pick your first projects carefully: To give your NLP initiative a greater chance of succeeding, you should start with a project that is relatively low risk. In other words, you shouldn’t start with your hardest problems, as these tend to have a higher chance of failure. For example, fully automating customer support may be too difficult, as that requires AI competencies outside of NLP. Instead, your first project should help build up your basic NLP capabilities and infrastructure. Projects like text classification or document categorization are comparatively simple, and can provide you with a firm base to build from.
4. Test and evaluate the performance of your models: Investing in AI models also requires an investment into their maintenance. You should regularly test and evaluate your models’ performance to make sure they are performing as expected. Regular evaluations can help you identify issues like model drift - changes in a model’s operational environment, or core variables that cause it to lose accuracy. In cases where model drift is present, you may need to retrain your models. Depending on the type of model, you may choose to continuously monitor its key performance indicators and retrain it when performance dips below a certain level, or retrain it at regularly scheduled intervals.
5. Ensure compliance with relevant regulations: The insurance industry is heavily regulated, and it’s important to make sure that your NLP models are used in compliance with relevant regulations, such as privacy laws and fair lending laws. You’ll need to give special attention to data protection regulations when training your models with user data, and work to eliminate any harmful bias in your training dataset. Taking preventative steps like these can help avert legal issues, and protect the interests of your business and customers.
Conclusion
The scope of possibilities enabled by NLP is remarkably wide, and can be tailored to meet the needs of each individual business. By analyzing and processing large amounts of data, NLP can empower your employees to make more informed and accurate business decisions. Additionally, automating burdensome tasks can free up time and resources to be dedicated to more pressing, or value-added activities. Enabling efficiencies of this sort are always going to be beneficial; whenever you can help your employees help your customers, you will reap the rewards twice over. Not only will you benefit from a more efficient workforce, but any efficiencies that help refine and expedite customer experience may also reward you with a more loyal customer base.
Want to know if an NLP solution is right for your insurance business? Schedule a free consultation with one of our expert data scientist’s here, and find out what we can do to help.
SOURCES:
Amaresh, K. (2022, May 17). How AI Adoption Can Impact the Insurance Industry. Forbes. https://www.forbes.com/sites/forbesfinancecouncil/2022/05/17/how-ai-adoption-can-impact-the-insurance-industry
IBM. (2018 March). Incumbents Strike Back. https://www.ibm.com/downloads/cas/KJDDERLW
Dewalt, K. (2021, November 13). The Coming NLP Revolution in Insurance. Prolego. https://www.prolego.com/blog/the-coming-nlp-revolution-in-insurance
Smolaks, M. (2019, August 16). Intelligent document imagine with natural language processing and optical character recognition. AI Business. https://aibusiness.com/nlp/intelligent-document-imaging-with-natural-language-processing-and-optical-character-recognition
Soberanis, L. (2021, September 13). From underwriting to claims management, artificial intelligence will transform the insurance industry. IBM. https://www.ibm.com/blogs/watson/2021/09/ai-will-transform-insurance-industry/
Paczolt, M. (2022, March 15). The complete guide to claims triage: Lowering workers’ compensation costs with predictive analytics. Milliman. https://us.milliman.com/en/insight/the-complete-guide-to-claims-triage-lowering-workers-compensation-costs-with-predictive-analytics
CallMiner. (2020). The CallMiner Churn Index 2020. https://assets.ctfassets.net/xj0skx6m69u2/6p1Z0Umw05scJuRvsS5hTl/447a9af2f959b4e1d7e6176c8ea5da3f/2020-callminer-churn-index-insurance-us.pdf
Kovalenko, K. Customer Churn Prediction and Prevention. BizData. https://www.bizdata.com.au/blogpost.php?p=customer-churn-prediction-with-algorithms#:~:text=Sentiment%20Analysis%20for%20Customer%20Churn%20Prediction,-Attempting%20to%20identify&text=There%20are%20two%20potential%20approaches,customer's%20voice%20during%20a%20call
Thematic. Sentiment Analysis: Comprehensive Beginners Guide. https://getthematic.com/sentiment-analysis/
MonkeyLearn. Guide to Aspect-Based Sentiment Analysis. https://monkeylearn.com/blog/aspect-based-sentiment-analysis/#:~:text=Aspect-based%20sentiment%20analysis%20
Coalition Against Insurance Fraud. 2020 Fraud Stats.
https://insurancefraud.org/fraud-stats/Federal Bureau of Investigation. Insurance Fraud Statistics.
https://www.fbi.gov/stats-services/publications/insurance-fraudAccern (2022, March 16). How AI is Transforming Insurance Claims Fraud Detection. https://accern.com/blog/how-ai-is-transforming-insurance-claims-fraud-detection/
Putatunda, J. (2020, June 10). Natural Language Processing (NLP) in Fraud Analytics. https://www.indellient.com/blog/natural-language-processing-nlp-in-fraud-analytics/
Contiamo. Intelligent insurance claims routing for a multinational casualty insurer. https://uploads-ssl.webflow.com/5b4d97b58ebe677e65f7ebf1/5c753263a272b12f6b80386a_Intelligent%20claims%20routing.pdf
Wilcox, K. (2022, August 9). Role of NLP in Claims Management. https://www.insurancethoughtleadership.com/claims/role-nlp-claims-management
Drenik, G. (2022, April 9). The Customer Experience Overhaul In Insurance. https://www.forbes.com/sites/garydrenik/2022/04/19/the-customer-experience-overhaul-in-insurance